Artificial Intelligence to discover simplified descriptions of complex phenomena
Automatically finding collective coordinates of a physical system by analyzing and optimizing their information content
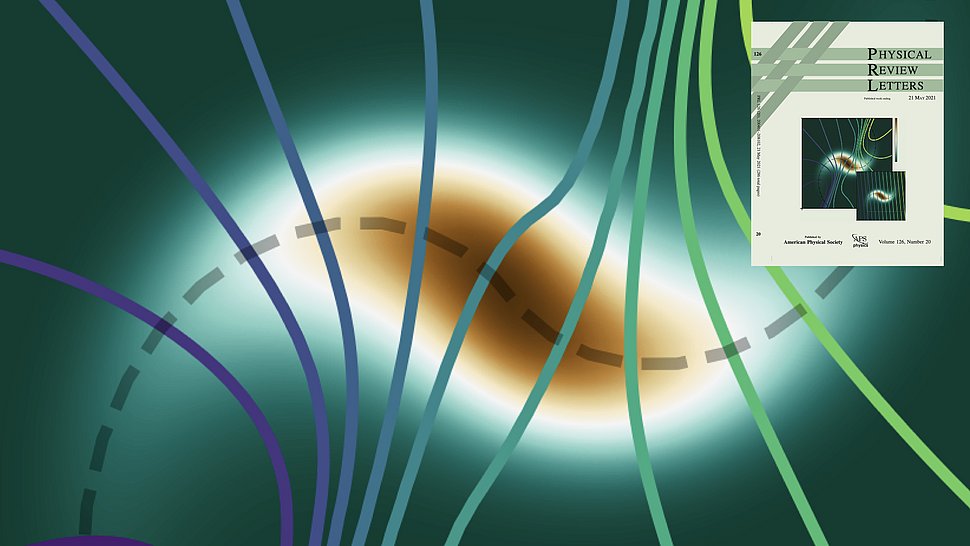
The process of scientific inquiry became much more powerful and efficient during the last fifty years because of technological advance: experiments are more precise and sophisticated and the results can now be stored and analyzed with the help of computers. Numerical simulations are now a crucial tool for the understanding of complex phenomena. However, how models and theories are created did not change much: they still totally rely on the intuition and understanding of the scientist. Considering that the essence of a scientific theory is to discover and exploit the regularities of a system to make predictions, there is no reason in principle why it should not be possible to automatically find these insights. This is the field of Artificial Scientific Discovery, where techniques that try to autonomously describe a phenomenon, without the explicit supervision of a human scientist, are explored. The development of these tools can potentially allow for a completely new wave of scientific discoveries in the next decades.
One of the most useful concepts in the analysis of a physical system is the notion of collective coordinates. In many cases, ranging from statistical physics to hydrodynamics, the description of a system can be dramatically simplified by considering only a few collective variables like the centre of mass, an order parameter or a flow field (think for example of solitons and optical vortices in the context of optics). However, in new situations, it is not clear a-priori which low-dimensional function is best suited as a compact description of the high dimensional data that the scientist observes.
In the work “Renormalized Mutual Information for Artificial Scientific Discovery” by Leopoldo Sarra, Andrea Aiello and Florian Marquardt from the Theory Division, recently published in Physical Review Letters, a technique to find these quantities was developed. The starting point is the use of statistics and information theory to “extract” the relevant quantities just by looking at many observations of the system. For example, consider a cloud of particles: if the quantity that changes most among different realizations of the system is the size of the cloud, then one can characterize it with its radius (and forget the position of all of the individual particles); if, on the other hand, they don't have a circular shape but are stretched in one direction, one should use the orientation angle of the cloud. In general, one looks for the low-dimensional description that provides the largest information about the system.
In the paper, the authors generalized the idea of mutual information, a solid concept in information theory, to work for this purpose, i.e. to quantify the amount of information between a random variable and a deterministic continuous function of it. In addition, by parametrizing these features and performing optimization of the conveyed amount of information on the system, they show that it is also possible to automatically extract collective variables.
This approach not only adds to the toolbox of artificial scientific discovery techniques, but can also find possible applications in the analysis of the information flow of neural networks and, in general, in representation learning techniques in computer science.
Original publication:
Leopoldo Sarra, Andrea Aiello, and Florian Marquardt
Phys. Rev. Lett. 126, 200601 – (2021). https://doi.org/10.1103/PhysRevLett.126.200601
Contact:
Leopoldo Sarra
leopoldo.sarra@mpl.mpg.de
Contact
Edda Fischer
Head of Communication and Marketing
Phone: +49 (0)9131 7133 805
MPLpresse@mpl.mpg.de