Learning how machines learn
Scientists from the University of Toronto and Mario Krenn from the MPL have developed a new method for observing how artificial neural networks process information and learn.
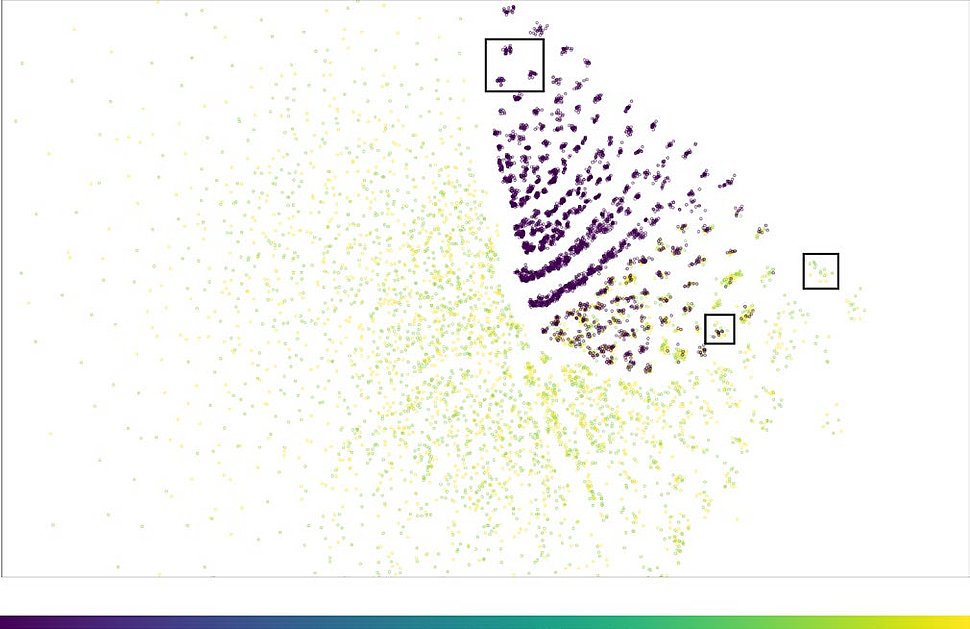
While the words artificial intelligence may conjure up imagines of science fiction movies where robots take over the world, in reality there have been many advances in recent years when it comes to creating „artificial brains“. Artificial intelligence is often driven by neural networks, that attempt to mimic the human brain. These can have varying numbers of layers, adding to the complexity of the system and its ability to categorize data and make predictions based on this. So called Deep generative models (DGM) are neural networks with multiple layers trained to approximate complicated probability distributions using large numbers of data samples.
Developing these DGMs has become one of the most hotly researched fields in artificial intelligence in the last few years. One advancement created with DGMs has even made it into public knowledge: the successes in generating realistic-looking images, voices, or movies – which has led to the development of so-called deep fakes. In more scientific approaches, deep learning methods have been used in chemical sciences for molecular design and for one of the most complex areas of physics: quantum mechanics.
Analyzing quantum mechanics with neural networks
Quantum mechanics include many phenomena that seem counterintuitive from a classical physics perspective. One example of this is quantum entanglement, which is a primary feature of quantum mechanics that is lacking in classical mechanics. One major complication with using DGMs and other neural networks is the lack of understanding for how they complete a task, how they get to a solution, how they learn. Without this understanding, scientists in turn cannot learn from the networks. That is why, in this paper, published in Nature Machine Intelligence, the authors wanted to create a model that would allow them to watch it learn.
The model in this paper called QOVAE (quantum optics variational auto encoder) was fed with a number of quantum optics experiments and given the task of reproducing those same experiments. In order to this, the network first had to understand the experiments it was given. The question here however, was not if the network was capable of this, but how it would go about doing it. This was what the researchers set out to try and observe. They achieved this by creating a sort of bottleneck in the neural network, where the network is reduced to 2 neurons, making it possible to view its inner workings in 2D.
The team, which includes MPL Research Group Leader Mario Krenn, found that QOVAE is able to sort experiments internally by specific measures like length of the experiment, device ordering or amount of entanglement, without having been told to do this, which enables it to better save the data. Now that it’s known, that this neural network thinks about experimental data like this, it may be used to design new quantum experiments without having to be trained. In Addition, this setup can now be used on more complex problems, possibly allowing researchers to learn new information from the internal processes of the network.
Picture 1: (Reprinted with the permisison of Daniel Flam-Shephard et al., Nature Machine Intelligence 4, 544-554 (2022)): The internal representation of quantum optics experiments in a neural network. Yellow (Violett): Experiments that do (not) create entangled quantum states. The discrete structure was a surprising outcome, and was understood as a clustering of similar experiments.
Contact:
Dr. Mario Krenn mario.krenn@mpl.mpg.de
Original Publication:
Daniel Flam-Shepherd, Tony C. Wu, Xuemei Gu, Alba Cervera-Lierta, Mario Krenn, Alán Aspuru-Guzik
"Learning interpretable representations of entanglement in quantum optics experiments using deep generative models", Nature Machine Intelligence 4, 544-554 (2022)
https://doi.org/10.1038/s42256-022-00493-5
Contact
Edda Fischer
Head of Communication and Marketing
Phone: +49 (0)9131 7133 805
MPLpresse@mpl.mpg.de