Artificial intelligence to accelerate the design of nanostructures
An artificial neural network helps to predict the band structure and topological properties of a metamaterial nanostructure.
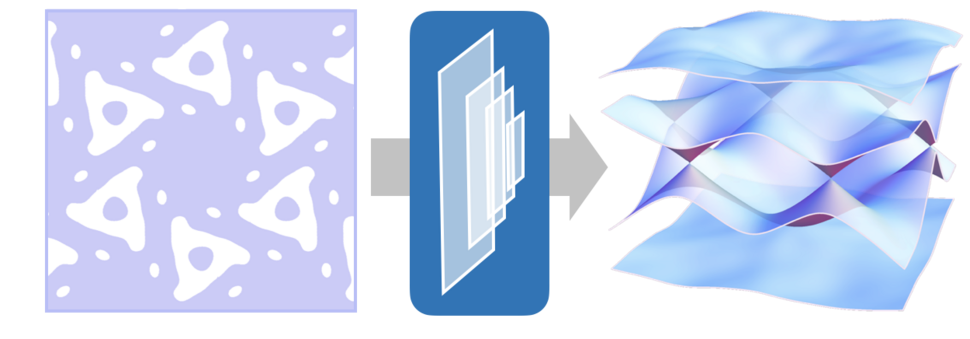
Graphic: MPL
The fabrication of arbitrarily patterned structures, down to the nanoscale, allows a large degree of control of the propagation of light and other waves in the resulting metamaterial. Most of the information about the propagation of a wave inside a regular structure, e.g. which light colours can propagate and at what speed, is condensed in a single mathematical object, the so-called band structure. The task of optimizing the fabrication pattern to achieve desired propagation properties is daunting because it involves exploration of the infinitely large space of all possible patterns, evaluating the band structure a large number of times.
In the work “Rapid Exploration of Topological Band Structures Using Deep Learning”, recently published in Physical Review X, Vittorio Peano, Florian Sapper, and Florian Marquardt from the Theory Division of the Max Planck Institute for the Science of Light in Erlangen have reported on their new deep-learning-based method to map arbitrary fabrication patterns to the corresponding band structures. This method involves a useful detour: Instead of directly predicting the band structure, a neural network learns to predict the parameters of an auxiliary “tight-binding” model that encodes not only the band structure but also the topological properties of the metamaterial. In a subsequent step, these properties can be accessed with an inexpensive computation based on the tight-binding model.
Thanks to the acceleration it provides for the optimization of band strucures, the deep-learning-based method developed in the Theory Division represents a promising tool to aid in the design of future nanostructures.
Original publication:
Vittorio Peano, Florian Sapper, and Florian Marquardt
Phys. Rev. X 11, 021052 – (2021). www.link.aps.org/doi/10.1103/PhysRevX.11.021052
Contact:
Vittorio Peano
vittorio.peano@mpl.mpg.de