Workshop on Artificial Scientific Discovery 2021
Please note that this website refers to a past event - for reference use only
This workshop aims to bring together some of the world's experts working on the most exciting routes towards true automated scientific discovery. The explicit purpose of this workshop is to emphasize those cases where the machine generates new insights on its own (rather than merely exploiting the well-known tools of machine learning as an aid in human-directed discovery). The most important goal of this workshop is to inspire discussions on possible avenues for future developments.
The workshop took place online, from June 29 (Tue) to July 1 (Thu), running each day from 4pm CET (=10 am EDT) to about 8pm, including a break.
Organizers
Leopoldo Sarra and Florian Marquardt (Max Planck Institute for the Science of Light, Theory Division)
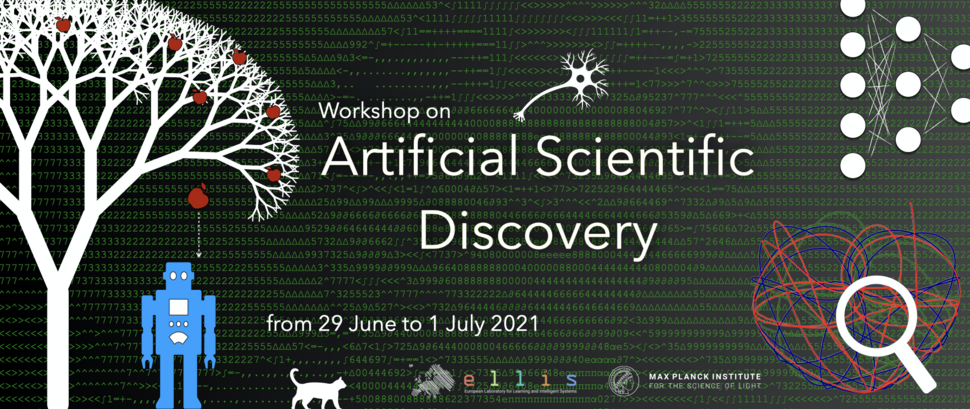
Scientific Program
Tuesday, June 29th
Introduction Recording
4:00 pm Ross King (Chalmers): The Automation of Science Slides Recording
4:40 pm Hans Briegel (Innsbruck): Projective simulation as an interpretable AI for basic science Slides
5:20 pm (break)
5:40 pm Natalia Ares (Oxford): From chips to qubits faster than human experts Slides Recording
6:20 pm James P. Sethna (Cornell): Sloppy models, differential geometry, and why science works Slides Recording
7:00 pm J. Nathan Kutz (U of Washington, Seattle): Deep Learning for the Discovery of Coordinates and Dynamics Slides Recording
Wednesday, June 30th
4:00 pm Renato Renner (ETH Zürich): Discovering physical concepts using neural networks Slides Recording
4:40 pm Mario Krenn (Toronto): A journey from computer-designed Quantum Experiments to computer-inspired Scientific Understanding Slides Recording
5:20 pm (workshop group picture and break)
5:40 pm Sebastian Wetzel (Perimeter Institute, Canada): Interpreting artificial neural networks in the context of theoretical physics Recording
6:20 pm Tailin Wu (Stanford): Machine learning of physics theories and its universal tradeoff between accuracy and simplicity Slides Recording
7:00 pm POSTER SESSION (open ended)
Thursday, July 1st
4:00 pm Kevin Ellis (Cornell): The Role of Higher-level Knowledge in Discovery Problems: Programs and Hierarchical Bayes Slides Recording
4:40 pm Georg Martius (MPI for Intelligent Systems, Tübingen): Discovering Equations using Machine Learning Slides Recording
5:20 pm (break)
5:40 pm Peter Battaglia (Deep Mind): Physical inductive biases for learning simulation and scientific discovery Recording
6:20 pm PANEL DISCUSSION and wrap-up (90 mins.)
Invited Speakers
- Natalia Ares (Oxford): From chips to qubits faster than human experts
- Peter Battaglia (Deep Mind): Physical inductive biases for learning simulation and scientific discovery
- Hans Briegel (Innsbruck): Projective simulation as an interpretable AI for basic science
- Kevin Ellis (Cornell): The Role of Higher-level Knowledge in Discovery Problems: Programs and Hierarchical Bayes
- Ross King (Chalmers): The Automation of Science
- Mario Krenn (Toronto): A journey from computer-designed Quantum Experiments to computer-inspired Scientific Understanding
- J. Nathan Kutz (U of Washington, Seattle): Deep Learning for the Discovery of Coordinates and Dynamics
- Georg Martius (MPI for Intelligent Systems, Tübingen): Discovering Equations using Machine Learning
- Renato Renner (ETH Zürich): Discovering physical concepts using neural networks
- James P. Sethna (Cornell): Sloppy models, differential geometry, and why science works
- Sebastian Wetzel (Perimeter Institute, Canada): Interpreting artificial neural networks in the context of theoretical physics
- Tailin Wu (Stanford): Machine learning of physics theories and its universal tradeoff between accuracy and simplicity
Contributed posters
Besides the invited talks, there will also be a virtual poster session, where we invite contributed presentations from anyone. Posters can cover a wide range of topics, as long as they are still related to machine learning or computer-enhanced discovery in the natural sciences and engineering.
- David Alonso González Oviedo, Universidad Autónoma Madrid: A deep learning approach to resonant light transmission through single subwavelength apertures
- Julian Arnold, University of Basel: Interpretable and unsupervised phase classification
- Thomas Chen, Academy for Mathematics, Science, and Engineering: GANs for chemobrionic modeling: exploring the origin of life
- Anshul Choudhary, North Carolina State University: Physics enhanced neural networks predict order and chaos
- Saso Dzeroski, Jozef Stefan Inst., Dept. of Knowledge Technologies: Probabilistic grammar-based equation discovery
- Lukas Fiderer, University of Innsbruck: Neural-network heuristics for adaptive bayesian quantum estimation
- Thomas Foesel, Max Planck Institute for the Science of Light: Quantum circuit optimization with deep reinforcement learning
- Doruk Efe Gökmen, ETH Zurich: Statistical physics through the lens of real-space mutual information
- Seungwoong Ha, Korea Institute of Science and Technology: Discovering invariants via machine learning
- Yaroslav Kharkov, Joint Quantum Institute, U Maryland: Machine learning of hydrodynamics in many-body quantum systems
- Annika Kreikenbohm, University of Wuerzburg: How immersive visualization can foster the cooperation of human and artificial intelligence
- En-Jui Kuo, University of Maryland: Decoding conformal field theories: from supervised to unsupervised learning
- Víctor López Pastor, Max Planck Institute for the Science of Light: Self-learning machines based on time reversal
- Shai Machnes, Forschungszentrum Juelich: An integrated tool-set for control, calibration and characterization of quantum devices applied to superconductive qubits
- Gorka Muñoz-Gil, ICFO Barcelona: The AnDI challenge: objective comparison of methods to decode anomalous diffusion
- Antonio Norelli, Sapienza University of Rome: Explanatory learning: beyond empiricism in neural networks
- Giambattista Parascandolo, MPI IS Tübingen & ETH Zurich: Neural symbolic regression that scales
- Vittorio Peano, Max Planck Institute for the Science of Light: Rapid exploration of topological band structures using deep learning
- Leopoldo Sarra, Max Planck Institute for the Science of Light: Renormalized mutual information for artificial scientific discovery
- Frank Schäfer, University of Basel: Control of stochastic quantum dynamics by differentiable programming
- Alessandro Simon, University of Tübingen & Max Planck Institute for Intelligent Systems: Learning classical density functionals
- Peter Tonner, National Institute of Standards and Technology: Interpretable modeling of genotype-phenotype landscapes with state-of-the-art predictive power
- Lea Marion Trenkwalder, University of Innsbruck: Intrinsically-motivated gadget mining for science
- Matthias Werner, Max Planck Institute for Intelligent Systems: Informed equation learning
- Hongbin Zhang, TU Darmstadt: CCDCGAN Inverse design of crystal structures
Poster submission deadline: June 25 (Friday) - please submit a link to a PDF of your poster (single page) to gesine.murphy@mpl.mpg.de , e.g. by uploading it to Dropbox, ownCloud, or a similar service.